Yimin Chen, Delving into differential privacy and anomaly detection: a meta-learning perspective
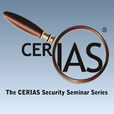
Summary: In this talk, we explore security and privacy related to meta-learning, a learning paradigm aiming to learn 'cross-task' knowledge instead of 'single-task' knowledge. For privacy perspective, we conjecture that meta-learning plays an important role in future federated learning and look into federated meta-learning systems with differential privacy design for task privacy protection. For security perspective, we explore anomaly detection for machine learning models. Particularly, we explore poisoning attacks on machine learning models in which poisoning training samples are the anomaly. Inspired from that poisoning samples degrade trained models through overfitting, we exploit meta-training to counteract overfitting, thus enhancing model robustness. About the speaker: Yimin Chen is now a postdoctoral researcher in Computer Science department in Virginia Tech. Currently his research mainly focuses on differential privacy, anomaly detection, adversarial example, and private learning. Before he worked on security and privacy of mobile computing systems for his PhD study. He obtained a PhD degree from Arizona State University in 2018, a MPhil degree from Chinese University of Hong Kong in 2013, and a BS degree from Peking University in 2010.