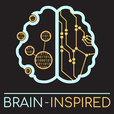
Summary: Randy and I discuss his LEABRA cognitive architecture that aims to simulate the human brain, plus his current theory about how a loop between cortical regions and the thalamus could implement predictive learning and thus solve how we learn with so few examples. We also discuss what Randy thinks is the next big thing neuroscience can contribute to AI (thanks to a guest question from Anna Schapiro), and much more. Computational Cognitive Neuroscience Laboratory.The papers we discuss or mention:The Leabra Cognitive Architecture: How to Play 20 Principles with Nature and Win!Deep Predictive Learning in Neocortex and Pulvinar.Unraveling the Mysteries of Motivation.His youTube series detailing the theory and workings of Leabra:Computational Cognitive Neuroscience.The free textbook:Computational Cognitive Neuroscience A few take-home points: Leabra has been a slow incremental project, inspired in part by Alan Newell’s suggested approach.Randy began by developing a learning algorithm that incorporated both kinds of biological learning (error-driven and associative).Leabra's core is 3 brain areas - frontal cortex, parietal cortex, and hippocampus - and has grown from there.There’s a constant balance between biological realism and computational feasibility.It’s important that a cognitive architecture address multiple levels- micro-scale, macro-scale, mechanisms, functions, and so on.Deep predictive learning is a possible brain mechanism whereby predictions from higher layer cortex precede input from lower layer cortex in the thalamus, where an error is computed and used to drive learning.Randy believes our metacognitive ability to know what we do and don’t know is a key next function to build into AI. Timestamps: 0:00 - Intro 3:54 - Skip Intro 6:20 - Being in awe 18:57 - How current AI can inform neuro 21:56 - Anna Schapiro question - how current neuro can inform AI. 29:20 - Learned vs. innate cognition 33:43 - LEABRA 38:33 - Developing Leabra 40:30 - Macroscale 42:33 - Thalamus as microscale 43:22 - Thalamocortical circuitry 47:25 - Deep predictive learning 56:18 - Deep predictive learning vs. backrop 1:01:56 - 10 Hz learning cycle 1:04:58 - Better theory vs. more data 1:08:59 - Leabra vs. Spaun 1:13:59 - Biological realism 1:21:54 - Bottom-up inspiration 1:27:26 - Biggest mistake in Leabra 1:32:14 - AI consciousness 1:34:45 - How would Randy begin again?