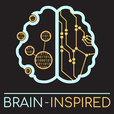
Summary: Support the Podcast Andrew and I discuss his work exploring how various facets of deep networks contribute to their function, i.e. deep network theory. We talk about what he’s learned by studying linear deep networks and asking how depth and initial weights affect learning dynamics, when replay is appropriate (and when it’s not), how semantics develop, and what it all might tell us about deep learning in brains. Show notes: Visit Andrew's website. The papers we discuss or mention: Are Efficient Deep Representations Learnable?A theory of memory replay and generalization performance in neural networks.A mathematical theory of semantic development in deep neural networks.A good talk: High-Dimensional Dynamics Of Generalization Errors. A few recommended texts to dive deeper: Introduction To The Theory Of Neural Computation.Statistical Mechanics of Learning.Theoretical Neuroscience.