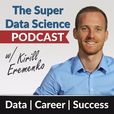
Summary: In this episode of the SuperDataScience Podcast, I chat with one of the key people behind the Python package scikit-learn, Andreas Mueller. You will learn about gradient boosting algorithms, XGBoost, LightGBM and HistGradientBoosting. You will hear Andreas's approach to solving problems, what machine learning algorithms he prefers to apply to a given data science challenge, in which order and why. You will also hear about problems with Kaggle competitions. You will find out the four key questions that Andreas recommends to ask when you have a data challenge in front of you. You will learn about his 95% rule to creating models, and creating success in business enterprises with the help of machine learning. And, finally, you will also learn about the Data Science Institute at Columbia University. If you enjoyed this episode, check out show notes, resources, and more at www.superdatascience.com/283